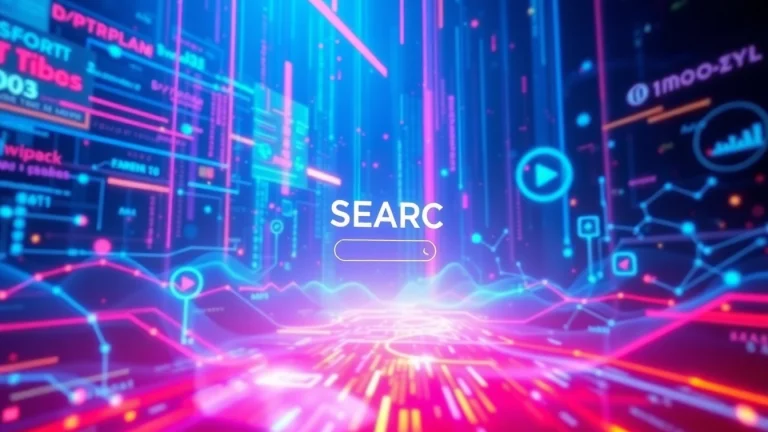
Maximizing Efficiency with AI Search: Techniques and Tools for Enhanced Discovery
Understanding AI Search: An Overview
Artificial Intelligence (AI) has revolutionized numerous fields, and search technologies are no exception. With the rise of AI search engines, users are experiencing a paradigm shift in how they access information. Unlike traditional search engines that retrieve data strictly based on keyword matching, AI search combines advanced algorithms with natural language processing to provide more relevant and contextual results. This article delves into the nuances of AI search, its workings, benefits, challenges, and how businesses can effectively implement such technologies.
The Evolution of Search Technologies
Search technologies have undergone a significant transformation since the advent of the internet. Early search engines were rudimentary, relying heavily on keywords and backlinks. For example, indexing was primarily about matching keywords with their appearances in documents. As the internet expanded, the need for more sophisticated solutions emerged. The introduction of algorithms like PageRank allowed search engines to rank pages based on quality and relevance, paving the way for modern search engines.
The integration of AI marks the next evolutionary step. AI search engines utilize vast datasets to learn and predict user behavior, making searching not just a retrieval process, but a more meaningful interaction. Users can engage with AI systems to receive tailored responses rather than a list of links, significantly enhancing user experience.
What Sets AI Search Apart?
At its core, AI search is distinguished by its ability to understand context, semantics, and user intent. Traditional search engines often return lists of links with varying levels of relevance, but AI search engines strive to provide direct answers or improve the relevance of search results through:
- Natural Language Processing (NLP): The ability to comprehend and process human language allows AI search to interpret queries with greater accuracy.
- Machine Learning: Continuous learning from user interactions helps improve the relevance of future search results.
- Contextual Understanding: AI can consider previous interactions and user preferences to tailor responses accordingly.
These features contribute to a more intuitive search experience, setting AI search apart from its predecessors.
Key Components of AI Search Engines
Several core components work synergistically to facilitate effective AI search functionalities:
- Data Inputs: High-quality, diverse datasets are crucial for training AI models. This includes both structured data (like databases) and unstructured data (like web pages and documents).
- Algorithms: Complex algorithms analyze patterns, learn from data, and predict probable outcomes, enabling the system to refine its results over time.
- User Interface: The design of the search interface is essential; it must be user-friendly and provide easy access to diving deeper into search topics.
- Feedback Mechanisms: Gathering feedback from users is vital for continuous improvement of search accuracy and relevance.
How AI Search Engines Work
The mechanics behind AI search engines involve a blend of technologies designed to enhance the overall search experience. Understanding this can provide insight into their effectiveness and potential applications.
The Role of Machine Learning in AI Search
Machine learning is at the heart of AI search engines. Through various techniques, such as supervised and unsupervised learning, AI systems can identify patterns and make informed predictions based on training data. For example, search models use machine learning to learn which types of responses users prefer based on their interaction history. This leads to refined search algorithms that can better predict user intent over time.
Data Algorithms: Parsing and Analyzing Information
Data algorithms play a crucial role in parsing, analyzing, and delivering information. They process vast amounts of data, identifying semantic relationships and contextual cues that inform the search results delivered to the user. Advanced algorithms, such as neural networks and decision trees, allow for nuanced data analysis that traditional keyword-based search methods lack. Furthermore, AI searches utilize techniques like content ranking and clustering to categorize and prioritize results effectively.
User Interaction and Feedback in AI Search
User interaction serves as both a measurement of success and a tool for improvement in AI search engines. Each query, click-through, and user response provides valuable data that AI systems utilize to evolve their models. Feedback loops create a system of continuous learning, allowing the engine to adapt and refine its accuracy. For instance, if users frequently adjust their queries or choose less advertisement-driven results, the search engine learns that the initial result ranking may need revising.
Benefits of Implementing AI Search
Adopting AI search technologies offers numerous advantages for businesses and consumers alike, fundamentally transforming search capabilities and the overall user journey.
Improved Accuracy in Search Results
With AI search, the accuracy of search results vastly improves due to the system’s ability to understand user intent and context. By analyzing past interactions and preferences, AI search can minimize irrelevant results, allowing users to find what they need faster and more efficiently. This is particularly beneficial in specialized fields like healthcare or legal services where precision in search results can have significant consequences.
Enhanced User Experience
AI search engines prioritize user experience through intuitive interfaces, direct responses, and personalized recommendations. Users no longer need to sift through pages of links; instead, they receive answers tailored to their queries. Consider services like Google Assistant or Apple’s Siri, which leverage AI to facilitate hands-free and conversational searching. This shift transforms the dynamic between user and technology, creating a smoother, more engaging interaction.
Real-time Data Processing and Insights
The ability to process and analyze data in real time is a significant advantage of AI search. Users can receive up-to-date information without the lag typically associated with traditional search engines. This is crucial in fast-paced environments such as finance or news, where timely access to data can inform decision-making. Additionally, real-time insights can assist organizations in adjusting strategies based on consumer behavior and trends identified through search activities.
Challenges in AI Search Technologies
Despite its advantages, AI search technologies face several challenges that must be addressed to fully realize their potential.
Data Privacy and Security Concerns
The integration of AI search necessitates the collection and analysis of user data, which raises potential privacy and security issues. Businesses must navigate regulatory landscapes such as GDPR and CCPA while ensuring that they’re transparent about their data practices. Implementing strong encryption protocols, conducting regular audits, and being clear about data usage can help build trust among users.
Overcoming System Limitations
AI systems are not infallible and may have limitations in understanding complex queries or niche topics. Ensuring that the AI has access to diverse datasets during the training phase is crucial; otherwise, the model risks developing biases or inaccuracies. Additionally, businesses should maintain fallback mechanisms that provide users with traditional search methods when AI search falls short.
Addressing Bias in AI Searches
AI searches can inadvertently perpetuate biases present within their training data. Consequently, ensuring fairness and neutrality in search results is paramount. Techniques such as regular model retraining, diversifying input data, and utilizing bias detection tools can help mitigate these issues. Organizations must remain vigilant about constantly evaluating their AI systems to prevent discriminatory outcomes.
Integrating AI Search into Your Business
For businesses looking to leverage AI search technologies, careful planning and implementation can make a significant difference in achieving desired outcomes. Below are steps for effective integration.
Choosing the Right AI Search Tool
Selecting an AI search tool that aligns with organizational needs is fundamental. There are numerous options available, ranging from open-source solutions to enterprise-level platforms. Evaluating features such as customization, scalability, and integration capabilities is crucial. Engaging stakeholders in this selection process can ensure that the chosen tool meets technical requirements and user expectations.
Best Practices for Implementation
Before rolling out AI search capabilities, businesses should establish best practices to streamline the process. These may include:
- Conducting thorough user training to mitigate potential resistance.
- Starting with pilot projects to test the effectiveness of the AI search tool before full-scale implementation.
- Regularly updating AI models with new data to maintain accuracy and relevance.
Such best practices not only enhance the chances of successful implementation but also foster a culture of acceptance and innovation within the organization.
Measuring Success: KPIs for AI Search
It’s vital to assess the performance of AI search solutions using Key Performance Indicators (KPIs). Useful metrics include:
- User Engagement: Track metrics such as click-through rates and session duration to gauge how users interact with search results.
- Search Accuracy: Monitor how often users find the information they seek on the first try.
- User Satisfaction: Gather qualitative feedback through surveys to assess overall satisfaction with search outcomes.
Regularly reviewing these KPIs allows businesses to make data-driven adjustments to enhance the AI search experience continuously.